The Best Image Labeling Tool: Transform Your Data Annotation Process
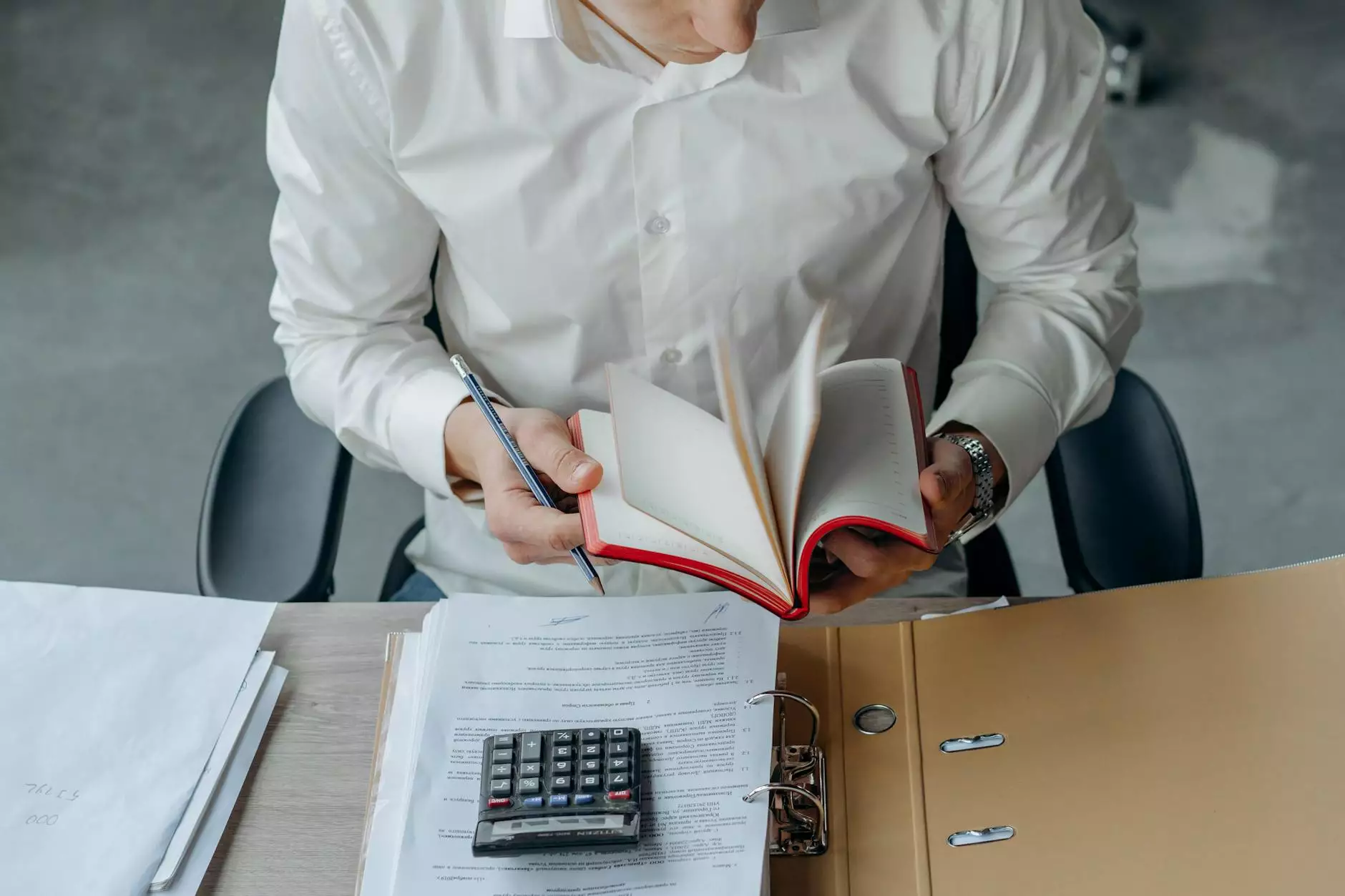
In the ever-evolving landscape of software development, the demand for high-quality data is paramount in training machine learning models. One of the foundational steps in this process is image labeling. As businesses increasingly rely on artificial intelligence and machine learning, the best image labeling tool is essential to ensure accuracy and efficiency in data annotation.
Understanding Image Labeling
Image labeling refers to the process of assigning tags or annotations to images, identifying objects, or classifying images for training machine learning models. This task is crucial for computer vision applications, including:
- Autonomous vehicles
- Facial recognition systems
- Medical image analysis
- Object detection in real-time applications
The rise in the need for accurate image labeling has led to the development of various tools designed to streamline this process. However, choosing the best image labeling tool requires an understanding of your specific needs and the features that can enhance productivity and quality.
Key Features of the Best Image Labeling Tool
When evaluating different image labeling tools, several key features should be taken into consideration:
1. User-Friendly Interface
A user-friendly interface is critical. It should be intuitive and allow users, regardless of their technical expertise, to easily navigate and use the tool effectively.
2. Support for Multiple Annotation Types
The best image labeling tools should support various annotation types such as:
- Bounding boxes
- Polygons
- Landmarks
- Semantic segmentation
This versatility enables users to cater to different project requirements seamlessly.
3. Collaboration Features
Collaboration is often essential in software development. Look for tools that allow multiple users to work simultaneously, comment, and review annotations in real time, thus improving overall productivity.
4. Integration Capabilities
The best image labeling tool should offer integration with popular machine learning frameworks and data storage solutions. This capability allows for a streamlined workflow where images can be directly fed into machine learning models for training.
5. Quality Assurance and Review Processes
A strong quality assurance mechanism is vital. The tool should provide options for revising and verifying annotations to ensure data quality, which is a significant factor in training accurate models.
Top Tools for Image Labeling in 2023
Here are some of the leading tools in the market that stand out as contenders for the best image labeling tool:
1. Labelbox
Labelbox is a powerful tool that offers an impressive array of features for image labeling. Users benefit from a collaborative platform with substantial annotation support, making it suitable for both small and large teams. Its automated labeling capabilities further enhance efficiency by speeding up the annotation process.
2. VGG Image Annotator (VIA)
The VGG Image Annotator is a free, open-source tool that offers straightforward annotation features. It is particularly favored for simple projects where a lightweight solution is needed. This tool supports various annotation formats, ensuring flexibility in usage.
3. Supervisely
Supervisely not only provides robust image annotation capabilities but also integrates project management features, making it ideal for teams that manage numerous projects. It supports various frameworks like TensorFlow and PyTorch, ensuring compatibility with machine learning workflows.
4. RectLabel
RectLabel is a paid tool primarily for macOS users, enabling high-quality image labeling with features like bounding box annotation and polygon drawing. Its simplicity makes it ideal for users focused on quick and efficient annotation without overwhelming complexities.
5. Roboflow
Roboflow excels in providing an end-to-end solution for image annotation and model deployment. It allows users to prepare their images, annotate them, and even train their models all within the same ecosystem, which is particularly advantageous for developers looking to streamline their workflows.
Best Practices for Effective Image Labeling
To maximize the efficacy of your image labeling efforts, consider these best practices:
1. Define Clear Guidelines
Establishing clear labeling guidelines is essential to ensure consistency across annotations. This is pivotal when multiple team members are involved in the annotation process.
2. Train Your Team
Invest in training your team members on the specifics of the labeling tool being used. A thorough understanding of the tool's capabilities can significantly improve the quality and speed of annotations.
3. Regularly Review and Evaluate
Implement regular reviews of the annotated images to ensure they meet your quality standards. Continuous feedback loops will help refine the skills of your team and enhance the overall outcome.
4. Leverage Automation
Make use of the automation features offered by the best image labeling tool you choose. Automated labeling can significantly reduce the workload for your team and expedite the annotation process.
Conclusion: Choosing the Right Image Labeling Tool for Your Business
In today's data-driven world, selecting the best image labeling tool is a critical decision that can influence the success of your machine learning projects. By understanding the key features and advantages of various tools, businesses can streamline their image annotation processes, enhance data quality, and ensure they are well-prepared for the challenges of AI and machine learning. Whether you're a startup or an established enterprise, investing in the right image labeling tool is essential for staying competitive in the software development landscape.
As you seek the perfect solution, keep in mind the importance of aligning tool capabilities with your specific project requirements and team dynamics. The right choice can lead to remarkable efficiencies and exceptional outcomes in your software development efforts.