Unlocking Business Potential Through Image Data Annotation
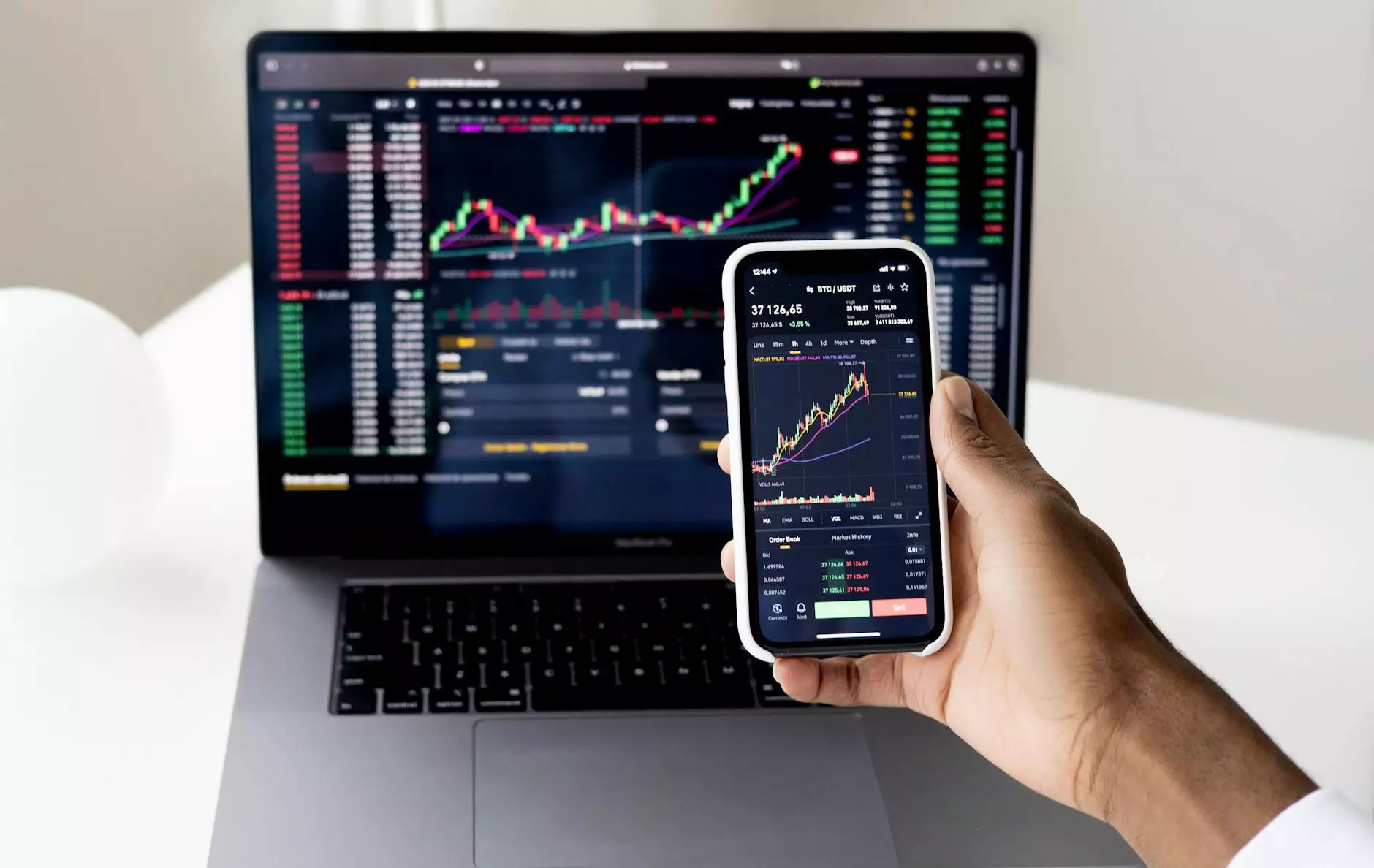
The digital landscape is evolving at an unprecedented pace, and businesses are increasingly relying on data-driven strategies to stay ahead of the competition. Among the myriad of data types, image data annotation plays a critical role in enhancing machine learning models, particularly in the fields of computer vision and artificial intelligence. This article explores the significance of image data annotation, the tools and platforms available, its impact on business processes, and best practices to ensure effective implementation.
What is Image Data Annotation?
Image data annotation refers to the process of labeling images for use in machine learning and deep learning applications, enabling algorithms to understand and interpret visual data. This labeling process is integral for training models to recognize objects, faces, or scenes in pictures effectively.
Data annotation enhances the capability of algorithms to draw insights and automate a wide array of tasks, including:
- Object Detection: Identifying and locating objects within an image.
- Image Segmentation: Dividing an image into segments to simplify analysis.
- Facial Recognition: Analyzing facial features for identification purposes.
- Activity Recognition: Tracking actions and behaviors in visual content.
The Importance of Image Data Annotation in Business
In an era where visual content dominates communication, businesses must leverage image data annotation to derive more value from their data. Here’s why it matters:
1. Enhanced Decision-Making
Companies utilizing annotated images can improve their decision-making processes. By training machine learning models with high-quality annotated data, businesses can gain insights into customer preferences, market trends, and operational efficiencies.
2. Improved Customer Experience
By deploying image recognition technologies powered by accurate image data annotation, businesses can refine customer interactions. For example, retail businesses can enhance their e-commerce platforms with image search capabilities, leading to a more personalized shopping experience.
3. Better Marketing Strategies
Marketing departments can leverage image data annotation to segment audiences more effectively based on visual content preferences, leading to tailored advertising strategies that resonate with target consumers.
4. Automation and Cost Efficiency
A well-annotated dataset enables easier automation of tasks, reducing labor costs associated with manual processes. Businesses can optimize resource allocation and drive efficiency.
Choosing the Right Data Annotation Tool
Selecting a suitable data annotation tool can significantly impact the quality and speed of your image data annotation projects. Here are some factors to consider:
1. Types of Annotations Supported
Different tools support various types of annotations. Ensure that the tool you choose can handle the specific annotations required for your use case, such as bounding boxes, polygons, or key points.
2. User Interface and Usability
A user-friendly interface can significantly reduce the time required for training annotators. Choose tools that offer straightforward navigation and tutorials.
3. Collaboration Features
Collaboration capabilities are crucial for teams working together on image data annotation tasks. Look for tools that facilitate real-time collaboration, allowing team members to provide feedback and make adjustments quickly.
4. Flexibility and Scalability
Your business needs may change over time. Select a tool that is adaptable and can scale with your growing data needs.
5. Cost & Support
Finally, consider your budget and evaluate the support options provided by the tool. High-quality tools may come at a premium, but they often deliver better results and support.
Exploring Data Annotation Platforms
Data annotation platforms offer a centralized environment for managing your annotation projects. Here’s a rundown of their benefits:
1. Streamlined Workflow
Data annotation platforms facilitate smoother project management by allowing users to organize, assign, and monitor tasks seamlessly.
2. Quality Control Mechanisms
These platforms often incorporate built-in quality control features to ensure that annotations meet predefined standards, thus enhancing the overall quality of the training data.
3. Integration Capabilities
Many platforms integrate with existing machine learning workflows or systems, enabling a more cohesive data pipeline.
4. Analytical Tools
Data annotation platforms may provide analytical tools to measure project progress, helping businesses make data-driven improvements to their processes.